Geometric Deep Learning for Computational Mechanics Part I: Anisotropic Hyperelasticity
Vlassis, Ma, Sun, CMAME, 2021.
We developed a machine learning approach that combines geometric deep learning and Sobolev training to generate a family of finite strain anisotropic hyperelastic models to predict the homogenized responses of polycrystals not seen during training. We use a weighted graph as a new high-dimensional descriptor to represent topological information, such as the connectivity of anisotropic grains in an assemble, and a graph convolutional deep neural network to extract low-dimensional features and learn the influence on the stored elastic energy functionals. We use Sobolev training to ensure smoothness and accuracy in predictions, and results from numerical experiments show improved predictions compared to classical training methods.
Read more →
Read more →
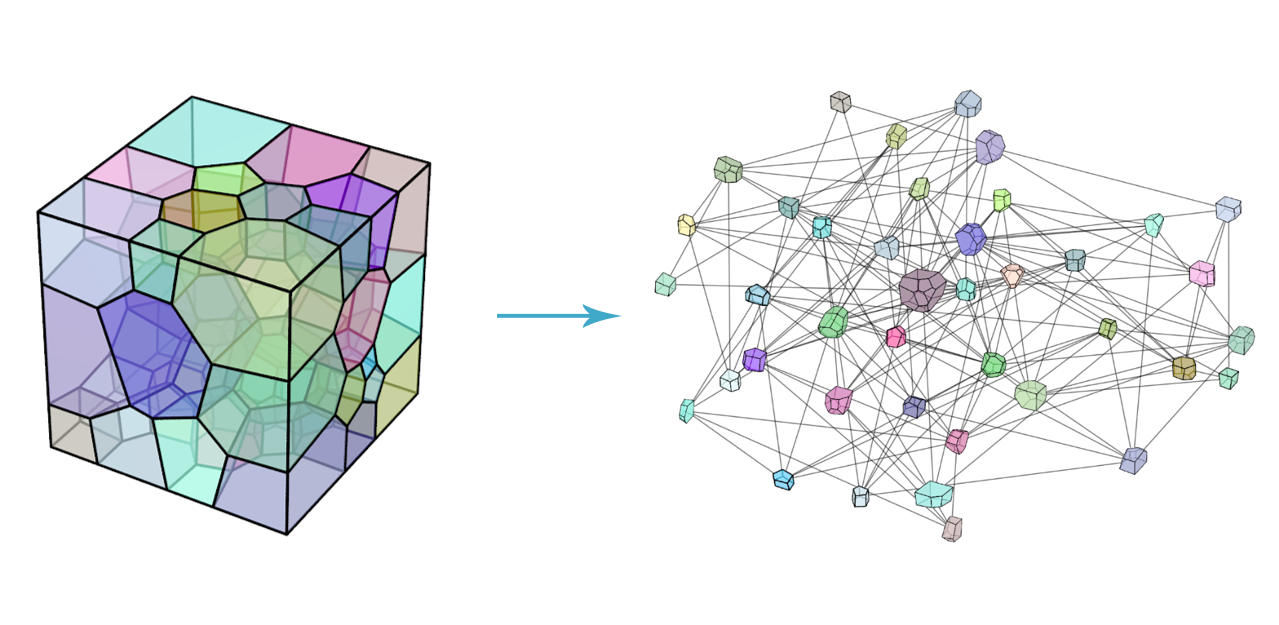
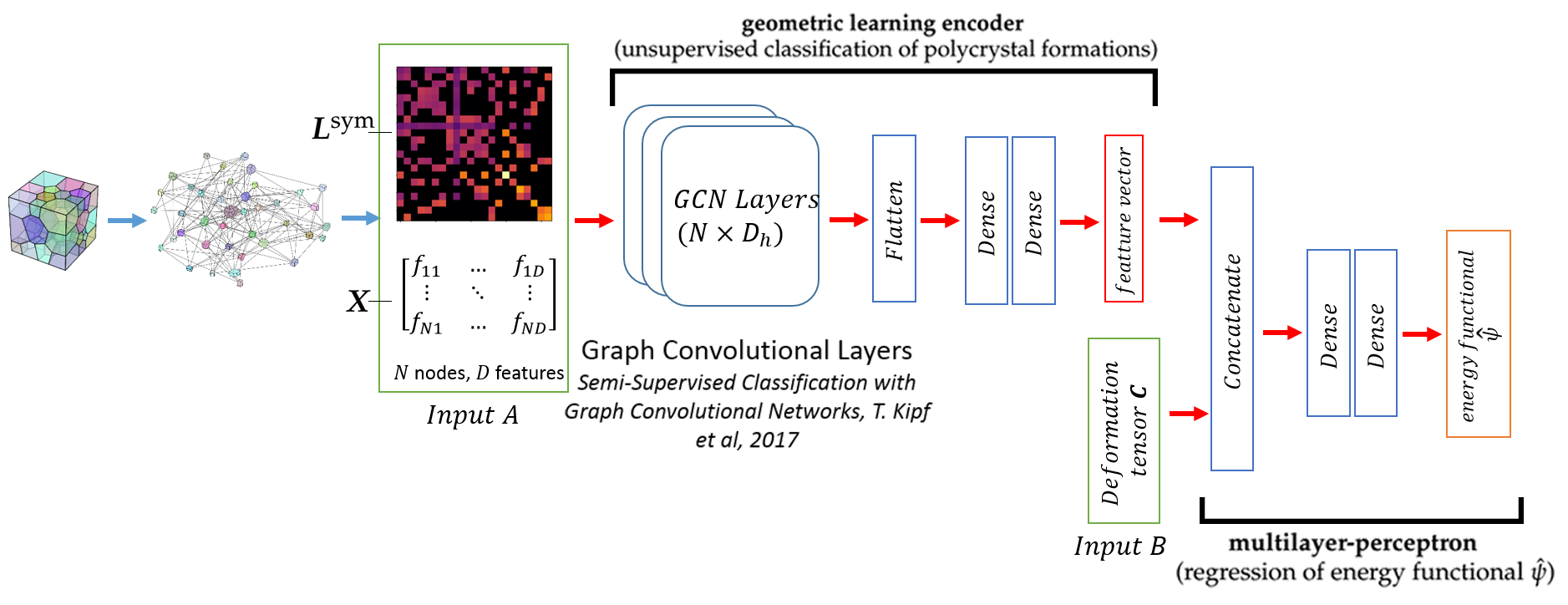
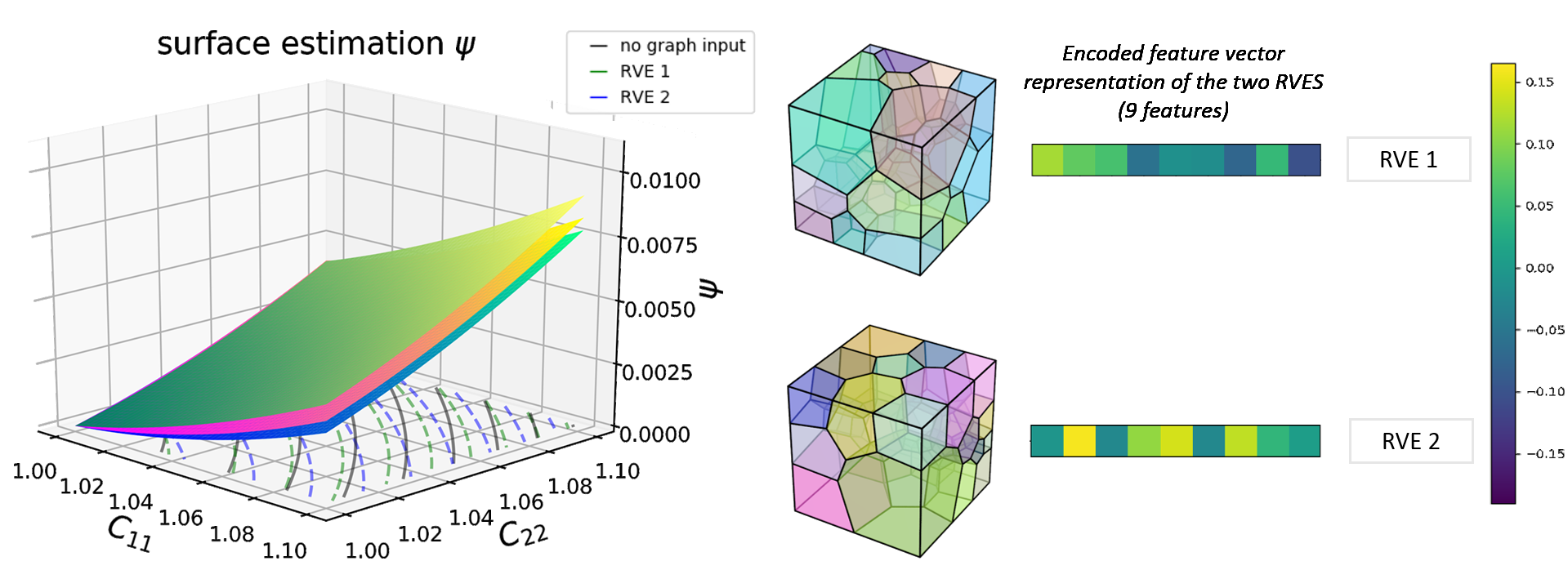